WEEK 7: Bias and ethics
(3) Objective.–The term `objective’, when used with respect to statistical activities, means accurate, clear, complete, and unbiased.” Sec. 3562 Coordination and oversight of policies, The Foundations for Evidence-Based Policy Making Act (1)
Goals
- Introduce the concepts of bias and fairness
- Introduce frameworks for understanding the risk-utility tradeoff
- Provide an overview of a practical toolkit for evaluating bias and fairness
The role of objectives and measures
In the realm of data science, two concepts have become increasingly important: bias and ethics. Understanding these concepts is crucial for policy analysts working with data and artificial intelligence (AI). Bias refers to systematic errors that can distort analytical results. Ethics refers to a set of principles and rules that guide decision making; those should ideally be open and transparent. Since policy implementation is inherently about allocating resources from one group to another group, someone gains and someone loses. Since there are no absolute criteria of what is fair and what is not, the National Academy of Public Administration has identified the need for a clear mission and quantifiable metrics as the primary principles for public organizations tasked with enhancing policy outcomes. Indeed, both the corporate world and the public sector require a governance structure that is designed to achieve a clearly defined objective (2, 3) and establishes the measures to gauge the success in achieving that objective (4). READ MORE
Bias and data ethics in practice
Bias can creep in at every stage of the data collection and analysis. In the early stages, the process by which data are generated may reflect existing inequalities. The collection and assumptions made in the processing of data may be biased. The training datasets that are developed for machine learning, which by definition reflect a sample of the population, can be a biased subset. The labelling of those datasets, which is often done by human classification, can reflect conscious or unconscious human bias. Finally the decisions that are made also be biased. (5) READ MORE
Ethical frameworks for evidence-based policy-making
There is a vast literature on what ethical frameworks are appropriate for producing evidence for policy-making. One of the most influential has been the Belmont Report which was set up in response to the 1932-72 US Public Health Service Syphilis Study at Tuskegee, developed a core set of principles: respect for persons, beneficence and justice (6).
In the federal context, Congress has long provided a framework for federal statistical agencies to produce high quality data as inputs for decision-making. Agencies are required to disseminate pertinent and timely information, carry out credible, accurate, and objective statistical activities. They are also tasked with safeguarding the trust of information providers by ensuring the confidentiality and exclusive statistical use of their responses. However, as the National Academies has pointed out, new approaches need to be developed(7). READ MORE
Balancing risk and utility
As noted in the previous section, the Evidence Act makes it clear that agencies must define clear user needs and public benefits, the use of minimally intrusive data and tools, and access to data to ensure accountability and transparency in methods, while also ensuring security (8). The Act also emphasizes that access is central to developing trust in how data are combined, identifying coverage issues – especially for underrepresented or vulnerable groups, particularly in the absence of common identifiers and usable metadata. Transparency and accountability are particularly critical in this context.
Paul Romer, the 2018 Nobel Laureate in Economics summarizes the importance of openness with great clarity.
“PR: We must promote open and transparent information sharing, including data production, and finance it. We need to change the incentive structure for information from one driven by pure profit to one driven by something more amenable to the academic model (people doing research because they are interested in sharing ideas). We should de-privatize the information industry—even go as far as putting Google out of business so that we can reduce concentrations of economic and political power as a result.” (9)
Introduce a practical tool-kit for evaluating bias and fairness
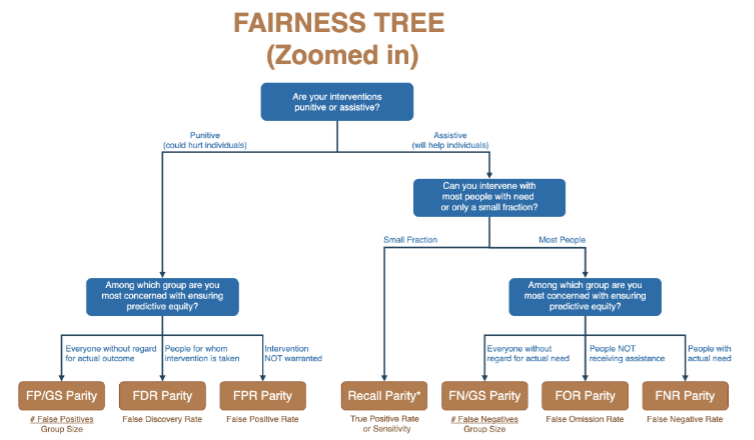
Source: Aequitas
Fairness is a concept central to ethical decision-making, can be a complex notion to define and measure, particularly because it can be subjective and context-dependent. In essence, fairness in data science and AI refers to the idea that decisions made by these systems should be equitable and just, not favoring one group over another based on characteristics like gender, race or ethnicity.
While it’s true that reasonable people can have differing opinions on what constitutes an ethical decision, there should be consensus on what needs to be measured and incorporated into decision-making processes. As Simon Winchester points out: “All life depends to some extent on measurement, and in the very earliest days of social organization a clear indication of advancement and sophistication was the degree to which systems of measurement had been established, codified, agreed to and employed”(10). In this context, tools like the AEQUITAS toolkit can be invaluable. This toolkit provides a robust framework to measure the biases in any decisions that are made, offering a practical way to operationalize and quantify fairness. READ MORE
Food for thought
There has been a flurry of activity in recent weeks around AI and ethics. READ MORE
Readings
- Bias and Fairness, Chapter 11 in the textbook
- Why they’re worried Student paper for Data Science in Context
- Data Ethics Presentation to Advisory Committee on Data for Evidence Building
- Aequitas: An open source bias toolkit (as much as you want to read)
Reference
- US Congress, editor Foundations for Evidence-Based Policy Making Act of 2018. 115 th Congress HR; 2018.
- Hand DJ. Aspects of data ethics in a changing world: Where are we now? Big data. 2018;6(3):176-90.
- Paine LS, Srinivasan S. A guide to the big ideas and debates in corporate governance. Harvard Business Review. 2019:2-19.
- Karpoff JM. On a stakeholder model of corporate governance. Financial Management. 2021;50(2):321-43.
- Bias and Fairness, Chapter 11 https://textbook.coleridgeinitiative.org/chap-bias.html (Sections 11.1 – 11.2)
- Spector, A. Z., Norvig, P., Wiggins, C., & Wing, J. M. (2022). Data science in context: Foundations, challenges, opportunities. https://datascienceincontext.com/manuscript/ (sections 3.2 and 3.3).
- National Academies of Sciences, Engineering, and Medicine. Federal statistics, multiple data sources, and privacy protection: next steps. 2018: 133-156.
- Advisory Committee on Data for Evidence Building. Advisory Committee on Data for Evidence Building: Year 2 Report Washington DC. 2022 Recommendations 1.2-1.9.
- Romer, P., & Lane, J. (2022). Interview With Paul Romer. Harvard Data Science Review, 4(2). https://doi.org/10.1162/99608f92.cc0da717
- Winchester S. The perfectionists: how precision engineers created the modern world: HarperLuxe; 2018.